Course: Human Factors in The Aviation/Aerospace Industry
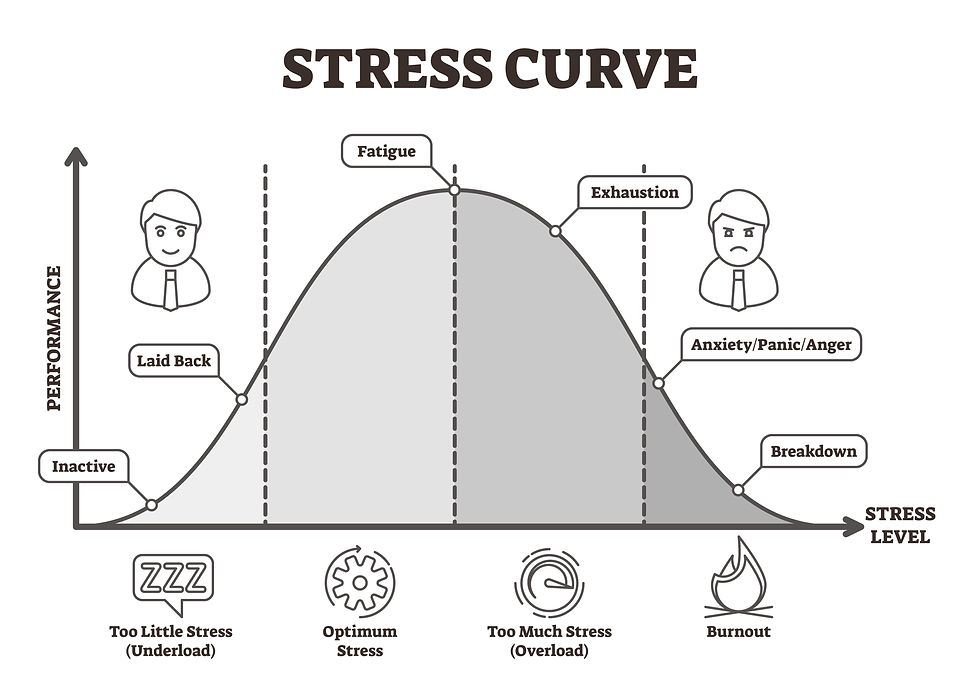
Abstract
An exponential rise in Unmanned Aerial Systems (UAS) usage is forecasted within the next decades. Regulatory agencies have been seeking to measure and mitigate potential human factors effects of increased UAS usage on air traffic controllers. Researchers anticipate mental fatigue, stress and pressure to be the main adverse effects of increased workload on air traffic controllers (ATC). This may consequently lead to poor decision making that is detrimental to the national airspace regulatory agencies performance and efficiency goals and objectives. To measure the potential effects of increased UAS usage on air traffic controllers, a within-subjects experimental research design shall be conducted to observe, measure and analyze the impact of increased workload due to the progressive rise in UAS usage. Upon completion of the research study, researchers shall establish correlations between increased air traffic controllers’ level of fatigue and increased UAS usage in the national airspace.
Introduction
Humans’ passion for flight fostered the design of advanced flying machines and the creation of the aerospace industry. Throughout this growth, technological advancement has taken center stage. The human factors field in aviation was essentially created due to the necessity of preventing adverse actions related to the use of advanced human-computer systems. It is focused on the safety of aviation personnel, aircraft, facilities, equipment and infrastructures. Its objective is to minimize adverse actions in aviation due to human errors. The main elements that led to these humans errors are complacency, fatigue and stress (Guastello, 2014).
Unmanned Aerial Systems (UAS) are the newest type of aircraft being designed and developed by aircraft manufacturers. Teal Group predicts worldwide military UAS production of ninety billion over the next decade (Teal Group Corporation, 2018). The Federal Aviation Administration(F.A.A.) forecasts total sales of hobbyist and commercial UAS to rise from 2.5 million in 2016 to 7 million in 2020 (F.A.A, 2016). With such a high number of UAS entering national airspace, what is the human factors effects of increased UAS traffic in the national airspace on air traffic controllers? In order to determine and establish answers to this question, an integrated research design shall be developed to measure the human factors impact of increased workload on air traffic controllers (ATC).
The potential impacts of UAS-based increased workload on air traffic controllers have been progressively studied by researchers and regulatory agencies. In their research on human systems integrations, Fern, Rorie and Shively warn that increased ATC workload may lead to a decrease of airspace efficiency due to the tendency of air traffic controllers to increase separation between aircraft and UAS in order to further prevent chances of adverse occurrences. Although tested experimental scenarios have yet to confirm such, the observation remains significant ( Fern, Rorie & Shively, 2014). The MITRE corporation reported that the use of different characteristics to define UAS routes has introduced a factor of complexity in analyzing flight plans and flight profiles. UAS(s) uses latitude and longitudes waypoints to define their flight paths and routes as opposed to the conventional uniquely named waypoints. This has significantly contributed to air traffic controllers’ exhaustion and mental fatigue (Kamienski & Semanek, 2015).
Methodology
A within-subjects experimental research design shall be conducted to measure the mental workload or level of fatigue and stress introduced by increased UAS usage on air traffic controllers. The target population shall be defined as a selected group of three hundred air traffic controllers composed of three to six air traffic controllers from each state. The minimum years of experience of each participant shall be set to two years. One hundred participants shall be selected from each of the following level of experience category : two to ten years, eleven to twenty years and twenty-one years to thirty-six years. Two research participants groups shall be created for this study. The first group will complete simulated air traffic control tasks using only aircraft flight paths data. The second group will complete simulated air traffic control tasks using both UAS and aircraft flight path data. The duration of each test shall be set to two hours.

Nine hundred aircraft flight paths data shall be available for the first test. It shall be a mixture of short distance flight and long-distance flight. Three hundred flight path shall be selected for each of the following categories : Flight duration less than two hours, flight duration between two hours and five hours and flight duration between five hours and more. For the second test, nine hundred aircraft flight paths and Nine hundred UAS flight path data shall be available for the test. UAS flight path data shall be progressively increased to reflect forecasted increase in UAS usage. The number of UAS flight shall slowly be increased during the second test using the following criteria: 100 UAS flight path data at test time T=0 min, 250 UAS flight path data at test time T= 30 min, 600 UAS flight path data at test time T= 60 min, 750 UAS flight path data at test time T= 90 min and 900 UAS flight path data at test time T= 120 min.

Participants for each group shall be selected randomly from each experience category. To mitigate ethical issues in the selection process, each participant shall pick a colored ping-pong ball from a non-transparent container evenly filled with red and blue balls for each participant experience category. It is important to note that in order to illustrate a within subject research design approach, each participant group shall take both tests one time.
An encephalogram device shall be used to measure and record brain activity data for each participant during each test. A computerized system which include a duration and quantity measurement software shall be used to measure the following variables for each participant during each test: number of aircraft and UAS flight paths computed, number of aircraft and UAS flight path computed for each flight length category and task completion duration.
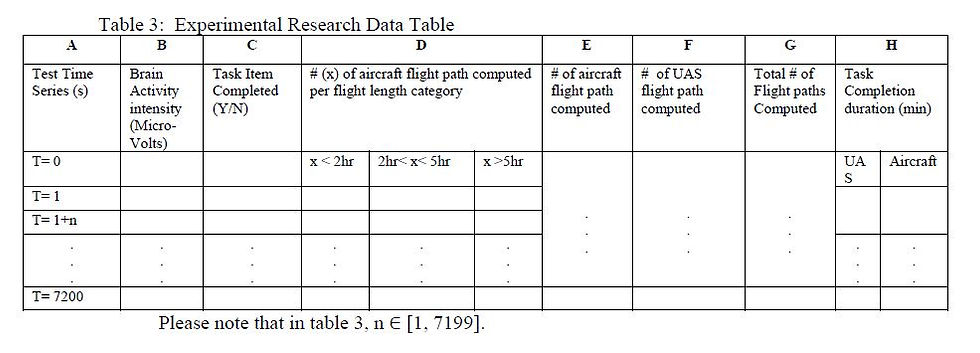
Results
Each group means shall be the research data used to compare differences between each measured group datasets. Measured brain activity data shall be used to determine the level of fatigue introduced by increased UAS usage on each traffic controller. A scatter plot of brain activity against number of flight paths computed shall be used to determine the set of data points that represent the threshold indicating overwork or mental fatigue. For each individual participant, this data point is the intersection point of the maxima of both variables. This data shall be computed for each test type. Researchers shall use a mathematical range analysis for each test type plots to determine the difference between the largest value and smallest value of each test type data sets. These numerical differences are a mathematical representation or quantification of the effects of increased UAS usage on air traffic controllers.
Using a basic matrix analysis, researchers shall also determine relationships between experimental variables. Researchers shall generate a correlation matrix table to illustrate the correlation coefficients between each sets of variables. A Variance and covariance matrix analysis shall be completed to illustrate how tasks completion duration varies based on the flight length category. This analysis shall be completed for brain activity and number of flight path computed, number of flight path computed and task completion duration, brain activity and total number of flight path computed including both UAS and aircraft. This data shall further demonstrate how each individual participant was affected by increased UAS usage. This provides a thorough insight into measured test variables and highlight relationship patterns between measured data sets.
The impact of increased level of fatigue on air traffic controllers shall be established through correlations between the measured level of fatigue introduced by increased UAS usage and tasks completion duration, number of flight path computed, and number of flight path computed per flight length category. A basic sets of scatter plots shall be used to highlight correlations ( if any) between the aforementioned variables. Researchers shall use the measured experimental data, determined relationships between research variables and the established correlations between increased brain activity and increased UAS usage to complete an impact assessment of the human factors’ effects of increased UAS usage on air traffic controllers.
Researchers shall establish a strong correlation between increased air traffic controllers’ level of fatigue and increased UAS usage. A basic scatter plot of fatigue level against number of computed UAS flight path shall suffice to illustrate the impact of UAS on air traffic controllers. It is important to note that a conclusion shall be made by researchers based on the measured level of fatigue caused by increased UAS usage and the efficiency of air traffic controller upon such a gradual increase of UAS usage.
Discussion
Measured experimental research data between test type 1 and test type 2 shall be compared. Any numerically positive data discrepancy between means of variables of test 2 and test 1 provide proofs or indications that increased UAS usage affects air traffic control activities. A numerically negative data discrepancy between means of variables for test type 2 and test type 1 simply indicated that there is no scientific proof of an impact of increased UAS usage on air traffic controller based on the experimental data and the research conducted.
Researchers shall focus on relationships and correlations between three variables: brain activity intensity, number of UAS flight path computed and tasks completion durations. On one hand, a positive correlation between brain activity intensity and number of UAS flight path computed will indicate that the increase in UAS usage leads to an increase of air traffic controllers’ workload. Such increased workload will lead to mental overload if no mitigation strategy is implemented. On the other hand, a negative correlation between brain activity intensity and number of UAS flight path will indicate that there is no proof of increased UAS usage having an adverse physiological impact on air traffic controllers.
Using the principle discussed above, a positive correlation between number of UAS flight paths computed and tasks completion duration will indicate that increased UAS usage has most likely introduced hardship or a level of complexity to air traffic controller assignments. Hardship equate to stress and pressure. This scenario highlights a potential impact of increased UAS usage on air traffic controller’s mental health. Although it is not sufficient to establish cause and effects, it does provide justification for the need of a true experimental research design to establish cause and effects. This shall also provide the root cause of this adverse action and establish that it is in fact solely due to an increased in UAS usage.
References
Federal Aviation Administration (2016, March 24). FAA Releases 2016 to 2036 Aerospace
Forecast. Retrieved from https://www.faa.gov/news/updates/?newsId=85227
Fern, L.R., Rorie, C.R., Shively, J. (2014, October 17). NASA’s UAS Integration into the NAS:
A Report on the Human Systems Integration Phase 1 Simulation Activities. Retrieved
Guastello, S. J. (2014). Human Factors Engineering and Ergonomics: A Systems Approach
(2nd Ed.) Boca Raton, FL: CRC Press of the Taylor & Francis Group.
Kamienski J., Semanek J. (2015). ATC Perspectives of UAS Integration in Controlled Airspace.
Procedia Manufacturing 3, P 1046-1051. Retrieved from https://doi.org/10.1016/j.promfg.2015.07.169
Teal Group Corporation. (2018, November 19). Teal Group Predicts Worldwide Military
UAV Production of $90 Billion Over the Next Decade [Press Release]. Retrieved from
https://www.tealgroup.com/index.php/pages/press-releases/56-teal-group-predicts-
worldwide-military-uav-production-of-90-billion-over-the-next-decade
Comments